As is common of NVIDIA’s GPU Technology Conference, this year’s event gave us a lot to think about, talk about, and get excited about. From deep-learning to super-fast graphics cards, the latest GTC had it all.
In this article, I am going to go over some of the biggest announcements and talked-about subjects that came out of the event, and also add in some quips about a few things that stood out to me personally.
Whenever NVIDIA CEO Jen-Hsun Huang takes the stage for a keynote, it’s rare when we know exactly what he’s going to say. At this year’s GTC, he didn’t beat around the bush: A core of all four announcements was deep-learning.
Deep-learning Craves GPUs
At 2014’s GTC, NVIDIA talked a bit about using GPUs for the sake of deep-learning, and overall, the concepts, implementations, and executions, were mind-blowing (and at times, mind-numbing, due to their complexities). At the most recent GTC, the amount of focus deep-learning received was major; as mentioned above, Jen-Hsun managed to tie it into all four of his announcements.
The reason for this keen focus shouldn’t come as a surprise: GPUs have proven to be excellent computational devices, and thanks to their massively parallel nature, they’re commonly an order of magnitude faster than traditional CPUs at things like deep-learning, where mass data is involved.
This ties into NVIDIA’s CUDA, which it first introduced in 2007 and began making a big deal about in 2008, in particular at its first-ever GTC – then called NVISION. During his presentation, Jen-Hsun highlighted the progress that CUDA has made since then; in 2008, there were 150,000 CUDA downloads, 27 CUDA apps, and 60 universities teaching GPU computing. In 2015, those numbers have soared to 3,000,000 downloads, 319 apps, and 800 universities.
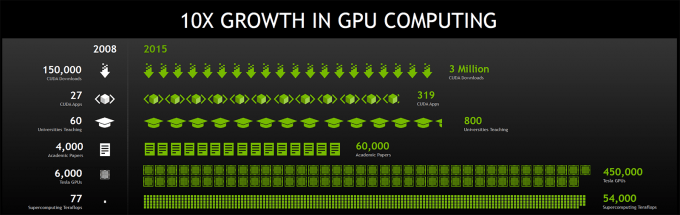
Perhaps the most staggering figures are those involving Tesla GPUs and overall performance. In 2008, 6,000 Tesla GPUs powered the world’s supercomputers, and delivered a total of 77 TFLOPs. Fast-forward to 2015, and the numbers boost to 450,000 GPUs and 54 petaflops.
Speaking of flops, NVIDIA launched its fastest GPU ever at GTC, called TITAN X. At this point, you’re probably already familiar with the card, especially as we posted a review of it last week, so I won’t rehash all of what it brings to the table here. However, tying into NVIDIA’s deep-learning focus, NVIDIA provides some special performance comparisons:
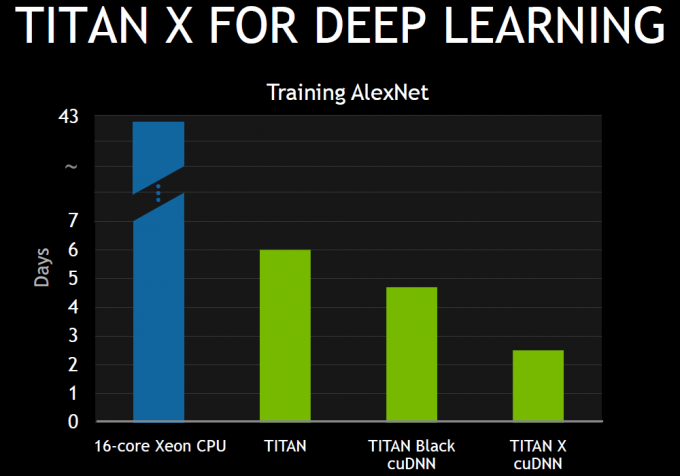
AlexNet is software that acts as a neural network to enhance the reliability of data classification. This is a perfect example of deep-learning; computers act like a brain (a neural network) to piece together a chain of bits of information in order to give an answer with a high rate of success. For example: a computer telling you the difference between a rottweiler and chocolate lab, or that there’s not only a car in an image, but that it’s parked next to a lake.
With that explained, you can look to the performance graph above to gain an understanding of just how much benefit a current TITAN X can offer a researcher versus the last-gen TITAN Black, and the TITAN before that. It’s almost hard to believe, given just how fast the original TITAN still is, but TITAN X can prove twice as fast two years later for the same cost. This graph also highlights why some data is better-suited for a GPU than a CPU.
To highlight the importance of deep-learning further, NVIDIA invited Google’s Jeff Dean and Baidu’s Andrew Ng to explain how they’ve both been using NVIDIA GPUs to accomplish some impressive things. Being that each keynote was over an hour-long and the subject of deep-learning is broad, I’d highly recommend you head here to watch them if you’re interested.
There are a couple of highlights I’d like to bring up, though, such as one that impressed me a lot during Jeff Dean’s presentation. Through the research of Google’s DeepMind group in London, computers taught themselves to play Atari video games, such as Space Invaders and Breakout. It works because the computers come to grips with the fact that they’re losing, and understand why. Then, because a computer has better reflexes than any human, it progressively becomes good enough where some games simply can’t be lost.
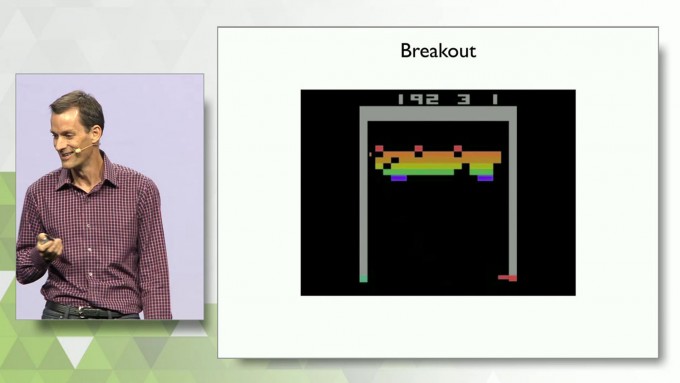
While voice-to-text technology is nothing new, Baidu’s Andrew Ng showed-off just how far it’s come. It’s not just about turning speech into written text anymore – it’s about doing so regardless of the environment. Examples were given where simulated crowd noise was played overtop of a speech block, and even in horrible conditions, the end result was quite good.
Autonomous driving played a big role during Jen-Hsun’s deep-learning keynote, and while it’s an area with a different kind of end-goal than those examples mentioned above, the learning is done in the exact same way. Why deep-learning is extremely important for something like autonomous driving is because if computations are not accurate, people’s lives will be at risk.
In an example shown, we were able to see how a mini-ATV called DAVE benefits from NVIDIA’s DRIVE PX – a number NVIDIA pits at 3,000x, compared to the original model.
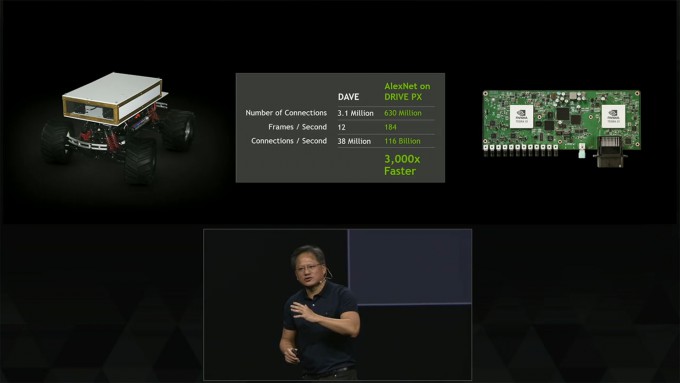
As with many of the examples above, DAVE had to teach itself how to drive through a complicated back yard. At first, the vehicle would run straight into the nearest roadblock; after a while, it understood which terrain and objects to avoid, and eventually made it to its end goal.
While this is a modest test, it’s the exact same kind of technology that feeds into our full-blown autonomous vehicles, and while things are impressive now, there’s still a way to go before autonomous driving can be considered ideal for real-world use.
In an interview with Tesla’s Elon Musk, a couple of interesting points about this are raised. For starters, Musk said that it’s not so much low-speed and high-speed (highway) that’s the big hurdle for autonomous driving, it’s mid-speed where autos will likely be passing through urban environments or crowded cities. It’s more challenging there simply because there are so many more factors at risk, with anything from someone at the side of a road about to get out of their car to an open manhole cover.
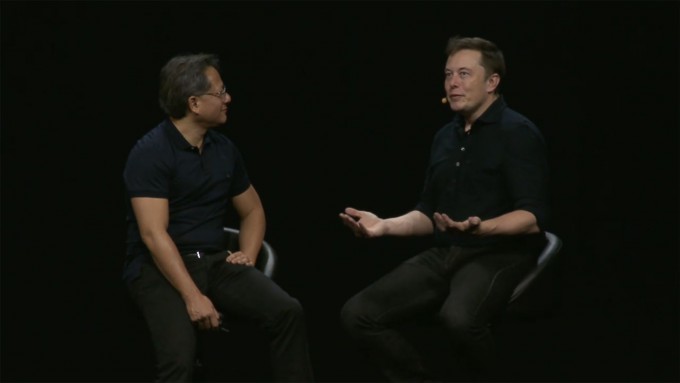
Musk fully believes that we’ll get there eventually, though; he even went as far to claim that in time, regular autos could be outlawed simply because autonomous will be so much safer and result in far decreased deaths. I am not sure I can see that ever becoming a reality myself, but it’s not hard to understand where he’s coming from.
Deep-learning computation isn’t something we’re going to be doing at home, but make no mistake: Its impact on different facets of our life can be substantial. It’ll be interesting to see where things stand at next year’s GTC.