The latest iteration of GTC (GPU Technology Conference) has just kicked-off, and for the third year in a row, it’s coming to us in the form of an online-only event. Fortunately, it’s free for everyone to sign up for and enjoy, with the only cost seemingly tied to special GTC Deep-learning Institute workshops, priced at $149. You can check out the official GTC website to register, or peruse an enormous list of available sessions.
GTC has become the de facto time of year for NVIDIA to really lay all of its wares on the table, with many new technologies and products unveiled. This year, the company has introduced a brand-new GPU architecture, named Hopper (after Grace Hopper), and to say it’s powerful would be a gross understatement.
Hopper has made its way into the H100, a new flagship AI GPU that sports 80 billion transistors, support for the new 4th-gen NVLink interconnect and 2nd-gen Multi-Instance GPU, has 4.9 TB/s of total external bandwidth, and is a chip built on TSMC’s 4N (4nm!) process.
NVIDIA’s CNX H100 GPU (SXM also available)
As with the previous generation, H100 is going to become available in either a PCIe 5.0 or SXM form-factor. Using NVLink, NVIDIA claims that 7x bandwidth can be delivered in comparison to the already really fast PCIe 5.0.
To help put things into better perspective, here’s where the new H100 stands in comparison to the flagships of the previous generations:
|
H100 |
A100 |
V100 |
P100 |
FP64 |
60 TFLOPS |
19.5 TFLOPS |
7 TFLOPS |
5.3 TFLOPS |
FP16 |
2,000 TFLOPS |
624 TFLOPS |
28.2 TFLOPS |
21.2 TFLOPS |
FP8 |
4,000 TFLOPS |
N/A |
N/A |
N/A |
TF32 |
1,000 TFLOPS |
312 TFLOPS |
112 TFLOPS |
N/A |
NVLink |
4th-gen |
3rd-gen |
2nd-gen |
1st-gen |
Memory |
HBM3 (80GB) |
HBM2e (80GB) |
HBM2 (32GB) |
HBM2 (16GB) |
Bandwidth |
3,000 GB/s |
1,935 GB/s |
900 GB/s |
732 GB/s |
TDP |
700W |
300W |
250W |
300W |
Because of how differently each new architecture may be used to tackle a certain type of computation, it’s difficult in some cases to compare one generation directly to the next. With A100, the FP16 performance is reflective of Tensor Core optimizations, which is why it towers over the performance of the Volta-based V100. That said, in this table, the H100 and A100 specs are truly apples-to-apples, resulting in the the new card being 3x faster than the previous generation for FP16 compute. The same gain applies to the TF32 Tensor performance.
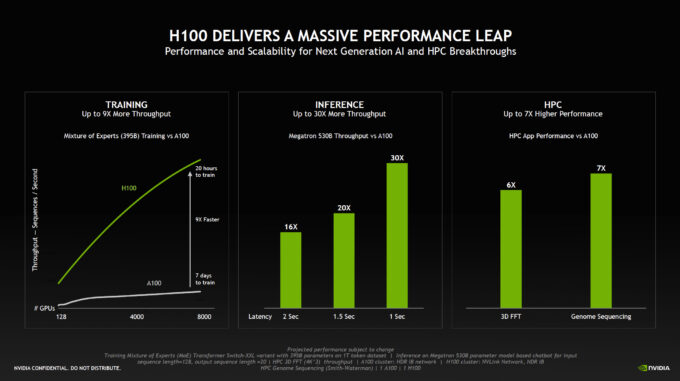
It’s not just FP16 that has seen such an enormous gain, but so too has FP64 double-precision. With Tensor core use, the A100 hit 19.5 TFLOPS, whereas that triples to about 60 TFLOPS in the H100. Without Tensor optimization, and based on the previous gen, this card would likely be spec’d at 60 TFLOPS FP32. NVIDIA hasn’t talked about that spec, because for the purposes of this card, it’s not particularly relevant. What it does tell us, though, is that this is one seriously fast, and capable architecture.
Specs tables sometimes only tell a part of the story, because in some cases, different tasks are going to benefit wildly different from others. With Hopper, one feature worth talking about is the new Transformer engine, which accelerates models that could be used for language, computer vision, medicine research, and so forth.
With a newly-added FP8 and Transformer Engine, advanced algorithms can combine its use with FP16 to crunch data as efficiently as possible. FP8 makes it possible to train larger networks easier, and while it seems FP8 could lack some accuracy of higher precision compute, that’s where the algorithm comes in. Where it makes sense to use FP16, it’s used, and vice versa for FP8. Calculations can be automatically re-casted and scaled between these layers. With the right scenario, NVIDIA says training can take days, instead of weeks.
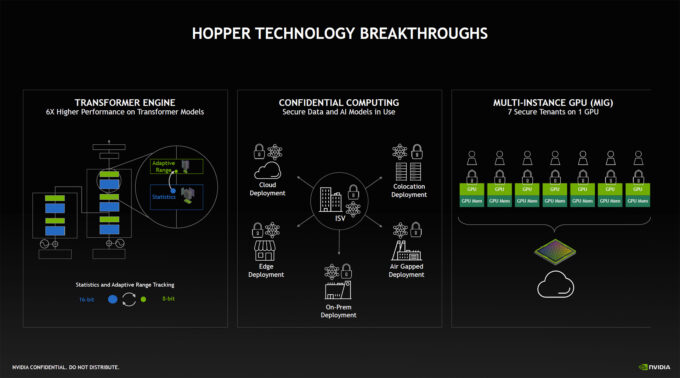
Three big “breakthroughs”, including the Transformer engine, are showcased in the above slide. The second point is Confidential Computing, a new design that allows in-use data to remain secure from every angle, so that code and data cannot be intercepted. NVIDIA notes that while models may be encrypted after-the-fact, in an effective rest state, that won’t prove to be a bullet-proof move if someone manages to gain access to the data as it’s in use. Considering how much money can be pumped into developing an advanced model, it’s clear why users would want to protect the goods.
NVIDIA notes that while it’s possible today to protect the data while it’s in use, it’s only CPU-based solutions that have existed, which are currently unable to handle the amount of work that a modern setup would demand. When using Hopper, a combination of hardware and software is used to create at trusted execution environment through a confidential virtual machine, that involves both the CPU and GPU. This sounds like something that could impede performance, an obvious no-no for such a deliberately high-end platform, but NVIDIA says the data still transfers at full PCIe speeds.
With the second-gen MIG (Multi-Instance GPU), NVIDIA expands on its technology that allows one GPU to be split up into seven segments, giving each segment to a user for them to get their work done with. What’s new here is added per-instance isolation, with I/O virtualization. This update feeds into the Confidential Computing addition, so security is really the name of the game here.
As became ultra-clear when NVIDIA acquired Mellanox, providing enough bandwidth for these powerful systems to transfer data around is crucial, and that’s why with each new generation of enterprise GPU, we seem to be greeted to an updated NVLink. New this year is NVLink Switch, a purpose-built network that can involve up to 256 GPUs, and delivers 9x higher bandwidth than NVIDIA’s own HDR Quantum InfiniBand.
Last, but not least, the H100 GPU also brings DPX instructions, useful to accelerate tasks like route optimization and genomics, by up to 40x when compared to CPUs, and 7x when compared to NVIDIA’s previous generation GPUs. Part of this includes the Floyd-Warshall algorithm which can be used for finding optimal routes for autonomous robots in a warehouse environment, and also Smith-Waterman, used for sequence alignment in protein classification and folding.
NVIDIA’s SMX H100 GPU (CNX also available)
With the launch of the H100, and Hopper architecture, we see NVIDIA continue to evolve its AI-focused GPUs to a significant degree, by not just improving performance all over the place, but also by adding new security features and overall capabilities. As the A100 was at the time of its launch, the new H100 is completely drool-worthy. Unfortunately for gamers, no gaming-related launch is taking place at GTC, but when you see the level of performance gained in this new AI GPU generation, it does bode well for NVIDIA’s future gaming chips.