With its just-announced Project Trillium, Arm wants to give the world useful artificial intelligence capabilities inside myriad of edge devices. Clearly, everyone and their dog wants a piece of the AI pie, but Arm is in an enviable place to deliver its solutions – its technology is already the de facto choice by an overwhelming number of mobile and IoT vendors.
Project Trillium (which is not the final branding, by the way) is a family of three separate components, two of which are processors. Those are the ML processor, for machine-learning purposes, and also OD, for object detection. The third component is Arm’s own NN (neural network) libraries which popular frameworks can be piped through.
WIth the ML processor, Arm says that it can deliver up to 4.6 TOPS (trillion operations-per-second) per chip, which equates to 3 TOPS/w. It’s not a fair competition given their vastly different market targets, but for comparison’s sake, NVIDIA’s Tesla P40 inference GPU hits 47 TOPS at 250W, giving us 0.188 TOPS/w. Granted, for that GPU, that TOPS performance comes in addition to 12 TFLOPS of single-precision, as well as 24GB of memory, so again, clearly very different markets here, but interesting nonetheless.
Arm supports all of the big deep-learning frameworks, such as Caffe, Caffe2, TensorFlow, MXNet, and Android’s NNAPI. With these, Arm covers the vast majority of the market, although on a call, the company noted that it’d be pretty trivial to support additional frameworks if the need came about, since most are very similar in design at their core.
As the shot above highlights, Arm’s neural network translates the framework piped into it, which allows developers to continue developing with their framework of choice as if the target platform didn’t matter. Arm will take over the translation duties, ultimately reducing the amount of time needing to be spent on tweaking to make sure things are just right.
The ML processor is going to be made available in the middle of this year, and while we’re not sure about all of the uses out-of-the-gate, the possibilities really are endless. As far as AI and deep-learning in general go, we’re still in early days. Given the momentum, and potential, it seems very likely that we’ll begin to see many more personal AI cases as the year goes on, either on our phones, or our security cameras (as a meager two examples).
One use case already revealed by Arm brings the second chip, the object detector, into the mix. With the OD chip, people inside of a scene would be quickly detected and monitored, which would then allow the ML chip to interpret what’s being seen to better adjust variables or simply gather information. A great example given by Arm is seen in the shot below. If a camera on a city street can monitor traffic, people, and even refuse bins, many things become possible. Instead of regular garbage pickups, for example, a camera would be able to monitor how much a bin is being used, and then only deploy someone to handle it when the need arises.
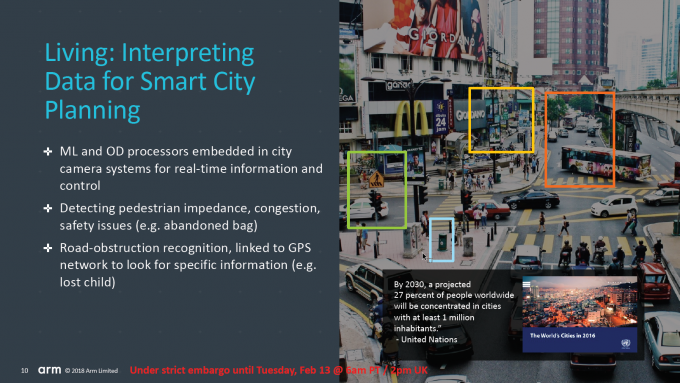
It should be noted that the OD processor here is actually a second-gen iteration. The first-gen was found in the Hive security camera, so the series already has a success story behind it. This second-gen chip supports full HD recording at 60 FPS, and the detection of objects as small as 50×60 pixels. It can also track things like standing positions, which makes this sound like a great solution for retail AR use, though the more boring but more important security aspect would make some great use of the technology, as well.
With the Mobile World Congress taking place in a couple of weeks, it wouldn’t be too surprising to learn of some Project Trillium design wins, and with the official launch for the ML IP being this summer, we could see the first supported devices this fall.