GTC has been running now for the last 5 years, with each annual event more expansive than the last. It’s a time for NVIDIA to bring together the top science, technology and creative professionals, and show them what’s currently in the works and where NVIDIA is going.
This year’s subjects are not surprising: Virtual and Augmented Reality, Autonomous Vehicles, 3D rendering, and the explosion that is deep learning. What is surprising is just the sheer growth in each sector, and this year saw some major advances coming down the pipeline.
For those that don’t know, GTC is not a consumer event, but more of a developer event. This means that most of the subjects brought up won’t have a direct impact on our daily lives, at least, not for the immediate future. This is a gathering for long-term commitments, as well as back-end development that trickles down to new products and services. However, that is not to say that everything is strictly business, as the first announcement will be of most people’s interest. NVIDIA’s new GPU architecture, Volta.
Volta: The Deep Learning Powerhouse
While NVIDIA only recently announced the last major updates to its Pascal line-up with the GTX 1080 Ti and TITAN Xp, The next big leap is already in development. However, it is worth pointing out here and now that a lot of what’s being shared won’t directly transfer over to the consumer cards, much the same as with the P100.
Volta’s first GPU announcement is the Tesla series compute card, the V100. To call it a beast of a card would be an understatement. Not only is it powerful with huge performance gains, a new compute core, and manufactured on the smallest node we’ve seen yet for a GPU, it’s also huge. At 21 billion transistors on a 815 mm² die, the chip is the size of the Apple Watch – at least according to Jensen Huang.
NVIDIA learnt a lot from Pascal, especially when it comes to deep learning. As such, Volta is an architecture built around fulfilling the desires of AI researchers and developers. Volta’s V100 is more than just a core count boost over Pascal, it adds a fundamentally new processing pipeline dedicated to deep learning with Tensor Cores. You can see the generation leap in the table below.
Tesla Product |
Tesla K40 |
Tesla M40 |
Tesla P100 |
Tesla V100 |
GPU |
GK110 (Kepler) |
GM200 (Maxwell) |
GP100 (Pascal) |
GV100 (Volta) |
SMs |
15 |
24 |
56 |
80 |
TPCs |
15 |
24 |
28 |
40 |
FP32 Cores / SM |
192 |
128 |
64 |
64 |
FP32 Cores / GPU |
2880 |
3072 |
3584 |
5120 |
FP64 Cores / SM |
64 |
4 |
32 |
32 |
FP64 Cores / GPU |
960 |
96 |
1792 |
2560 |
Tensor Cores / SM |
NA |
NA |
NA |
8 |
Tensor Cores / GPU |
NA |
NA |
NA |
640 |
GPU Boost Clock |
810/875 MHz |
1114 MHz |
1480 MHz |
1455 MHz |
Peak FP32 TFLOP/s* |
5.04 |
6.8 |
10.6 |
15 |
Peak FP64 TFLOP/s* |
1.68 |
2.1 |
5.3 |
7.5 |
Peak Tensor Core TFLOP/s* |
NA |
NA |
NA |
120 |
Texture Units |
240 |
192 |
224 |
320 |
Memory Interface |
384-bit GDDR5 |
384-bit GDDR5 |
4096-bit HBM2 |
4096-bit HBM2 |
Memory Size |
Up to 12 GB |
Up to 24 GB |
16 GB |
16 GB |
L2 Cache Size |
1536 KB |
3072 KB |
4096 KB |
6144 KB |
Shared Memory Size / SM |
16 KB/32 KB/48 KB |
96 KB |
64 KB |
Configurable up to 96 KB |
Register File Size / SM |
256 KB |
256 KB |
256 KB |
256KB |
Register File Size / GPU |
3840 KB |
6144 KB |
14336 KB |
20480 KB |
TDP |
235 Watts |
250 Watts |
300 Watts |
300 Watts |
Transistors |
7.1 billion |
8 billion |
15.3 billion |
21.1 billion |
GPU Die Size |
551 mm² |
601 mm² |
610 mm² |
815 mm² |
Manufacturing Process |
28 nm |
28 nm |
16 nm FinFET+ |
12 nm FFN |
Manufactured on TSMC’s 12nm FinFET process, it’s pushing the limits of photo lithography and quantum mechanics. However, it’s likely the 5120 CUDA cores that will have you sitting up with intrigue. Using the same HBM2 memory, the V100 is still capped at 16GB of VRAM, but with a whopping 900GB/s transfer rate. Computation of 15 TFLOPS peak FP32 (32-bit floating point), 7.5 TFLOPS FP64, are all nice and well-meaning, but it’s the 140 TFLOPS of Tensor Core computation that just puts the card in a league of its own.
Tensor Cores are a fundamentally new way to handle deep learning computation workloads, and requires a lot more than what this article can explain. They are effectively a massively parallel, low-accuracy, simple math engine used frequently in deep learning training. The performance boosts compared to Pascal are, to put it mildly, shocking. A 12x reduction in training time (research) and a 6x reduction in inference time (production) over Pascal.
Of course, the V100 is hardly going to be cheap, nor will it be released until Q3 this year. You will only see the V100 in pre-built systems that NVIDIA will be selling for the time being; the DGX-1 4U servers fitted with 8x V100s. Those systems will set you back a rather hefty $149,000, but NVIDIA does bring up some interesting points, namely system density.
A single DGX-1V has the equivalent performance of 800 CPUs. In one example, a 500 node CPU server costing $1.5m could be condensed into a 33 node GPU system, or increase the performance of the existing rack space by 15x. To be fair, those sorts of CPU only systems are rare, even in cloud computation. While Tensor calculations are not ‘common’ as a normal workload, they are becoming increasingly important in the world of virtual assistants like Cortana and Alexa – both of which perform millions of deep learning powered natural language database look-ups per second. Both Microsoft and Amazon are looking at Volta with glee.
Not everyone can afford such a beast of a server though, with researchers demanding something a little smaller they can pop under the desk without the supporting infrastructure to worry about (not to mention noise). It should be no surprise that even NVIDIA’s own research teams have been asking for something smaller, which is where the DGX Station comes in. The 4x V100 workstation will be the first ‘desktop’ deep learning station available that will likely have TITAN Xp owners feeling a little jealous. The $69,000 workstation still comes at a steep price, but considering that it can perform training runs in a matter of hours compared to days on Pascal cards, it’ll likely be money well spent. Since it isn’t server mounted, cooling had to be rethought and as such, the system is water-cooled, although it does require a 1500 PSU.
Deep Learning For Creative Industries
Deep learning isn’t just about empowering our new AI overlords, self-driving cars, and research. There are creative applications for the technology, some of which are rather CSI in nature with digital enhancement. We’ve seen some examples in the past with NVIDIA showing off its AI fueled upscaling engine which adds details back into a picture when increasing the resolution.
There is also a rather interesting application with a new wave of effects filters. Making your photos look like something out of a 70s Polaroid with bad lighting is one thing, but transferring over the ‘style’ of a famous photographer is another. In the GTC Keynote demonstration, one photo landscape style was transferred over to another, maintaining the details of the original, including the clouds, beach and sea, but artfully applied the coloring techniques of another photo over the top, creating a hybrid of the two.
Jensen went on to detail how deep learning could teach itself to differentiate between artists and apply techniques to others, or even tell if a painting is the real deal based on other works. However, most interesting of all, and something I’m sure a lot of creative professionals will be taking a very close look at, is image reconstruction and ray trace enhancements.
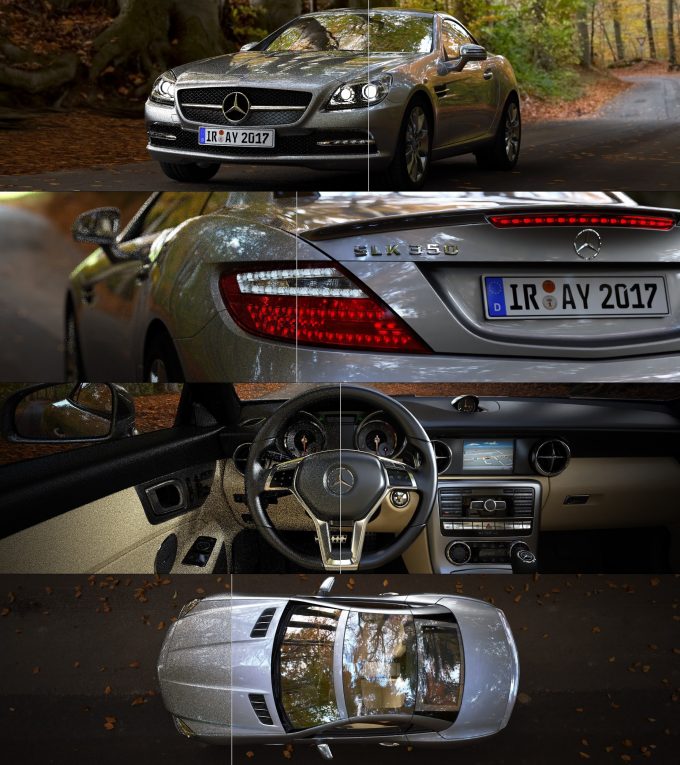
Although fairly early on in the presentation, one demo caught our attention as it’s something we’ve toyed with in the past, and that’s NVIDIA’s Iray renderer. In the demo, two scenes were being rendered in real-time using the iterative design process used by Iray, however, one of them had a deep learning AI tacked on top. The AI effectively guesses how the scene will render to fill out the details faster in a process called denoising using an autoencoder. The result is a production render appearing 4x faster than if left to run through the normal ray trace process. This is not exclusive to Volta, but is something that will be rolled out to Pascal-based Quadros. This deep learning functionality will be included in the Iray SDK later this year, where it’ll be integrated into applications and plugins that use Iray, including Mental Ray.
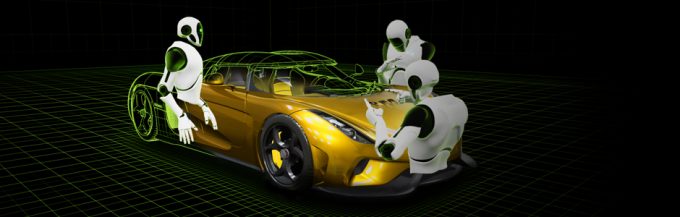
Iterative design is something that can significantly improve production speed and getting designs out the door for customers, but nothing quite beats real-time, instantaneous collaboration, and that’s where NVIDIA’s Holodeck comes into play.
Built around Epic Games’ Unreal Engine 4, Holodeck uses a mix of GameWorks, VRWorks and DesignWorks to build an interactive environment that multiple team members can then import and interact with 3D models. The GTC demo showed off a Koenigsegg Regera supercar, with over 50 million polygons being rendered and explored by four design team members.
Meet ISAAC – The Virtual Robot Simulator
Robotics has been an industry that goes through long phases of inspiration and stagnation. One of the major issues is teaching robots how to interact with their environments. Teaching them to perform the same task over and over is simple, but teaching them to perform the same task with variability in each step becomes tricky.
For a long time, humans had to teach robots how to account for that variability, but as soon as they are used outside of a known action, they fail, miserably. Teaching robots by hand is a painfully slow process, and an industry in desperate needs of assistance. Wouldn’t it be nice to teach a robot to teach itself how to perform a task? That’s precisely what deep learning is about.
A problem quickly arises, teaching a single robot to teach itself takes a very long time. What if you could teach hundreds or thousands at a time, and merge the best traits of all of them, then copy that AI over to each unit? That’s where ISAAC comes in, as it’s a virtual, physically modeled environment designed to teach robots how to perform certain actions. As a designer, you model the robot, give it a goal, and then watch as hundreds of iterations perform the same task, learning from mistakes as it goes.
As layers of tasks are built up, it possible to teach robots new tasks in a matter of minutes instead of days or months. When that action is finished, switch over to a new task. Rapid turnover of small tasks becomes possible without the need for expensive retraining.
NGC – NVIDIA GPU Cloud
Further reinforcing the deep learning development cycle, NVIDIA will be releasing its own cloud-based system called NGC. This allows developers and researchers iterate faster over a wide variety of hardware, from local servers, scaling up to cloud infrastructure.
By using a Docker approach to building pre-configured framework environments, developers can pick a build on a specific piece of hardware and have everything already setup and optimized, ready to start work. Each month, new versions of frameworks will be added, all kept up to date without the need to manage it yourself.
Workstations and servers on the company network can be setup as target points, or even GPUs on the existing rig. Once testing is complete, inferencing can then be started on a cloud-based production server operated by NVIDIA and scaled as demand grows. The main benefit from this is the always up-to-date frameworks optimized for the hardware; you can even choose specific builds of each framework.
The sheer growth in the deep learning sector is truly astonishing. While there are few products that make use of it locally in the consumer sense, it powers the AI behind the products, such as Alexa, Google Home, and Cortana.
With new processing enhancements, software could see a major boon when it comes to efficiency, or risk being left behind. There are thousands of startups based on AI research. While creative industries may not be at the forefront of deep learning, it’s still capturing some attention. Needless to say, autonomous vehicles and robotics are the two to watch out for. Be prepared to see some of the fastest product turnarounds in history over the next year or two.